数学模型预测模型
The NFL season is right around the corner. Players are nearing the end of training camp, and fans alike are eager to watch their favorite team play on Sunday again. How we got here has been unconventional to say the least. The offseason has been one we’ve never seen before, as the same can be said of this year in general. We should all be thankful there will even be a 2020 season given how this year has played out. But never less football appears to be back, and everyone is getting ready.
NFL赛季即将来临。 球员们即将接近训练营,球迷们都渴望再次在周日观看他们最喜欢的球队比赛。 至少可以说,我们如何到达这里是非常规的。 休赛期是我们从未见过的休赛期,与今年的情况一样。 鉴于今年的表现,我们都应该感恩,甚至会有2020赛季。 但是,足球似乎再也没有回来,每个人都在准备。
有钱可赚 (There’s Money to be Made)
When I say everyone is getting ready I’m talking about; coaches, players, fans, sports outlets, sports writers, sports betters, and Vegas. Vegas may look like the odd one out but I ensure you they have prepared for the 2020 season more than anyone else. Why is that you may ask? Well because their in the industry of information. The more information they have the more money you lose.
当我说每个人都在准备时,我是在谈论; 教练,球员,球迷,体育用品店,体育作家,体育用品和维加斯。 拉斯维加斯可能看起来很奇怪,但我确保您比其他任何人都为2020赛季做更多的准备。 为什么会问? 好吧,因为他们从事的是信息产业。 他们拥有的信息越多,您损失的钱就越多。
See as the 2020 season rolls around Vegas will put odds out on hundreds of events that will happen in the current season, and every year millions of betters lay money on those odds hoping they occur. Spoiler Alert! Many of those betters will lose. Why? Because those odds don’t just come out of thin air. No, they are generated by elaborate models that when inputted information will output a probability of an event that most betters will often over or under value. This is why most betters lose. They simply don’t have the info that Vegas has.
拉斯维加斯将在2020年赛季如火如荼地进行,这将使本赛季将要发生的数百场赛事的赔率有所提高,而且每年都有数百万名优胜者为希望发生的那些赔率提供资金。 剧透警报! 这些优胜者中的许多都将失去。 为什么? 因为这些可能性不只是凭空产生的。 不,它们是由精心设计的模型生成的,这些模型在输入信息时将输出发生事件的可能性,而大多数情况下,发生这些事件的可能性往往会过高或过低。 这就是为什么最好的人会输掉的原因。 他们根本没有维加斯拥有的信息。
There are those that will win against Vegas and continually win against them. These betters have created their own models that compete with the ones Vegas uses, and is where our famous model comes into place.
有些人会赢得拉斯维加斯的胜利,并不断赢得他们的胜利。 这些更好的人创建了自己的模型,可以与Vegas所使用的模型竞争,这就是我们著名的模型得以应用的地方。
著名模特 (The Famous Model)
Your probably wondering what this quote on quote “Famous Model” is, well it was created by Nate Silver, the founder and editor in chief of FiveThirtyEight and his team.
您可能想知道引文“ Famous Model”的引文是什么,它是由FiveThirtyEight的创始人兼主编Nate Silver和他的团队创建的。
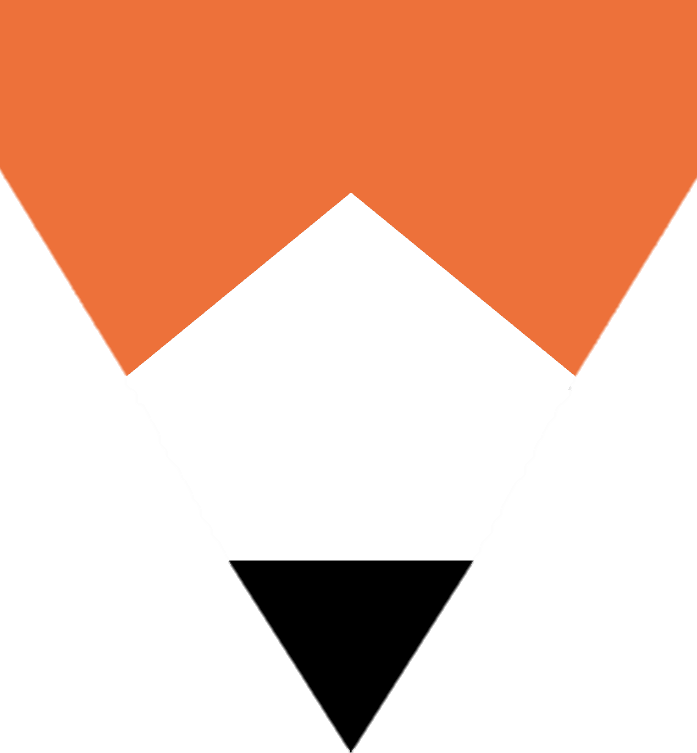
FiveThirtyEight is a website focused on politics, economics, and sports blogging. There famous for using statistical analysis and hard numbers to tell compelling stories and create models that show or predict an outcome. Questionably their most famous is their NFL prediction model. The model has been seen to compare to those in Vegas and is the reason many professional sports betters use it. They rely on the predictions and bet accordingly to what the model says.
FiveThirtyEight是一个专注于政治,经济学和体育博客的网站。 那里以使用统计分析和困难数字来讲述引人入胜的故事并创建显示或预测结果的模型而闻名。 无疑,他们最著名的是他们的NFL预测模型。 该模型已被证明可以与维加斯模型相提并论,这是许多职业体育爱好者更好地使用它的原因。 他们依靠预测,并根据模型所说的进行相应的下注。
The model was created in 2014 and has been continuously updated over the years to further improve the accuracy of the predictions. But in 2020 I have found ways to improve the model and increase the accuracy of the predictions even further. I will go more into detail later on but I think it’s time you finally learn what this famous model is and how it works.
该模型于2014年创建,多年来不断更新,以进一步提高预测的准确性。 但是在2020年,我已经找到了改进模型和进一步提高预测准确性的方法。 稍后我将进一步详细介绍,但我认为是时候该终于了解这种著名的模型及其工作原理了。
埃洛 (Elo)
If your familiar with how players are ranked in chess then your familiar with Elo ratings. Elo rating is a simple system that judges teams or players based on head-to-head results, and is the core of the model created by FiveThirtyEight. These Elo ratings are able to forecast the outcome of every game and generate win probabilities for each team. The nuts and bolts of this system are described below.
如果您熟悉棋手的排名方式,那么您将熟悉Elo等级。 Elo评分是一个简单的系统,可以根据正面结果来判断球队或球员,并且是FiveThirtyEight创建的模型的核心。 这些Elo评分能够预测每场比赛的结果并为每支球队产生获胜概率。 该系统的螺母和螺栓如下所述。
游戏预测 (Game predictions)
To begin with, the Elo model assigns every team a power rating. Those ratings are used to generate win probabilities for games based on the difference between the two team’s Elo ratings. Teams increase their Elo rating by winning games and decrease by losing, but by how much depends on the expected result of the game.
首先,Elo模型为每个团队分配功率等级。 这些等级用于根据两队Elo等级之间的差异生成游戏的获胜概率。 球队通过赢得比赛来提高Elo等级,而通过输掉而降低,但是幅度多少取决于比赛的预期结果。
For example, lets take two teams and give them each an Elo rating. Lets say the Dallas Cowboys are versing the New York Giants. The Dallas Cowboys have an Elo rating of 1650 and the New York Giants have a rating of 1400. Since Dallas’s rating is higher we know they have won more games than New York and should be favored in this game. In fact I can tell you, the Elo model gives them a win probability of 80%.
例如,让两个团队为每个团队分配Elo等级。 可以说达拉斯牛仔队正在与纽约巨人队对抗。 达拉斯牛仔队的Elo等级为1650,纽约巨人队的等级为1400。由于达拉斯的等级较高,我们知道他们赢得了比纽约更多的比赛,因此在本场比赛中将受到青睐。 实际上,我可以告诉您,Elo模型为他们提供了80%的获胜概率。
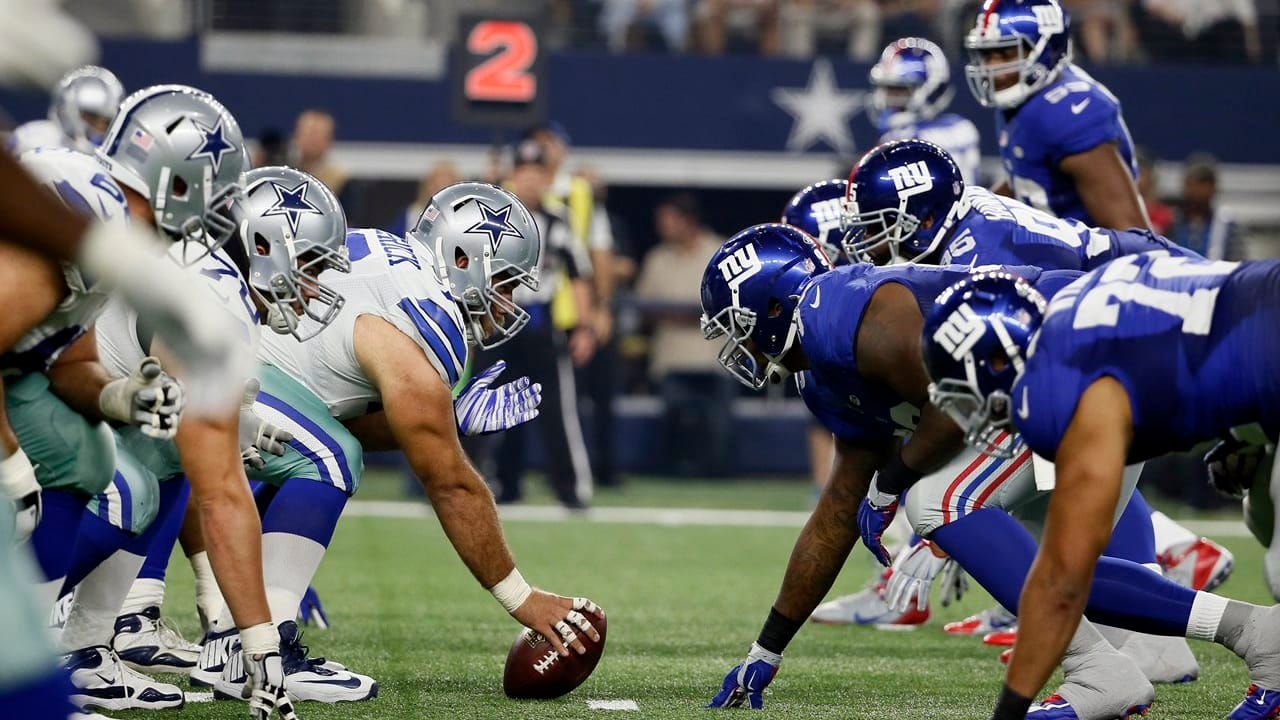
The reason Elo ratings are called a head-to-head system is because if Dallas were to win this game they would take points from New York, meaning their Elo rating would increase by how much New York’s decreases. Determining that number depends on the expected result. Since Dallas is favored to win by a large margin, if they do win their Elo rating will only increase about 5 points. However if New York wins, their Elo rating will increase by roughly 17 points. Elo takes in the level of the opponents and rewards more to the underdogs and less to the favorites. This is to ensure teams with high Elo ratings are winning against teams of their caliber and not just against below average teams.
Elo等级之所以被称为对头系统,是因为如果达拉斯赢得这场比赛,他们将从纽约获得积分,这意味着他们的Elo等级将增加纽约的跌幅。 确定该数字取决于预期结果。 由于达拉斯倾向于大幅度获胜,因此如果他们赢得胜利,他们的Elo评分只会增加大约5分。 但是,如果纽约获胜,他们的Elo评分将提高大约17点。 Elo接受对手的级别,对弱者奖励更多,对最爱的奖励更少。 这是为了确保Elo评分较高的团队在与其同等水平的团队中取得胜利,而不仅仅是在低于平均水平的团队中取得胜利。
调整项 (Adjustments)
On the surface the Elo system is very simple. If a team wins a game their Elo rating increases based on the expected outcome, vice versa if they were to lose said game. But football is more complicated then that. Elo ratings show the overall strength of the football team as a whole, but what it doesn’t take in is the outside circumstances that affect a football game. Circumstances that include: is the team playing at home or away, are they coming off a bye week, or are there changes at starting quarterback.
从表面上看,Elo系统非常简单。 如果一支球队赢了一场比赛,他们的Elo评分会根据预期结果提高,反之亦然,如果他们输了该局。 但是足球要复杂得多。 Elo评分显示了整个足球队的整体实力,但是没有考虑到影响足球比赛的外部环境。 情况包括:球队是在主场还是客场打球,他们是在再见一周之后出战还是在四分卫开始时发生变化。
There has to be adjustments for these situations to make sure the model is as accurate as possible. Therefore, FiveThirtyEight’s Elo model also includes several adjustments that take place before the start of each game:
必须针对这些情况进行调整,以确保模型尽可能准确。 因此,FiveThirtyEight的Elo模型还包括在每个游戏开始之前进行的一些调整:
Home-Field Adjustment — Teams in the NFL play better at home than away. In fact over the last 10 years, teams that play at home win by an average of 2.2 points more than if they were away. Therefore the model adjusts for this home field advantage by increasing the home team’s Elo rating by 55 points before the game.
调整主场-NFL的球队在主场比在远队更好。 实际上,在过去的10年中,在主场比赛的球队比不在场的球队平均赢得2.2分。 因此,该模型通过在比赛前将主队的Elo评分提高了55分来调整这一主场优势。
Rest Adjustment — Every team in the NFL gets 1 bye week (except for top-seeded teams that don’t play during opening week of the playoffs) and research shows that they play better than expected from their standard Elo alone coming off that bye week. To adjust for this better play, a team coming off a bye week gets an increase of 25 Elo points for that game.
休息调整-NFL中的每支球队都获得了1个再见周(除了在季后赛首周不参加比赛的顶级种子球队),研究表明,他们在标准再见周结束后的表现要比他们预期的Elo更好。 为了适应这个更好的比赛,一个小组在再见一周后获得了25点Elo积分。
Playoff Adjustment — Playoffs are very different from the regular season. In the playoffs it’s found that favorites tend to outplay underdogs by a wider margin. To adjust for this, the model increases the win probability of the favorite slightly in the playoffs.
季后赛调整 -季后赛与常规赛有很大不同。 在季后赛中,人们发现喜欢的人往往比弱者更胜一筹。 为了对此进行调整,模型会在季后赛中稍微增加收藏夹的获胜概率。
Travel Adjustment — Travel can have a huge affect on players from jet lag to being in different time zones. It has also shown to affect how teams as a whole play. Teams that have to travel across the country play a tad worse then one’s just a bus ride away. To adjust for this, the home team gets an increase of 4 Elo points for every 1000 miles the opponent travelled. This means the Giants get a 55 point Elo bonus when “hosting” the Jets (there’s no travel adjustment because they play at the same field, the 55 Elo bonus comes from the Home-Field Adjustment), while if Seattle comes to play, the Giants would get a 62-point Elo bonus for the large distance that Seattle had to travel.
差旅调整-从时差到不同时区,差旅会对玩家产生巨大影响。 它也显示出会影响整个团队的表现。 不得不穿越全国的车队的表现要差一点,而仅需乘搭公共汽车就可以了。 为了对此进行调整,主队每增加1000英里,对手就会获得4个Elo点。 这意味着巨人在“托管”喷气机时获得55点Elo奖金(由于他们在同一场比赛,所以没有旅行调整,55 Elo奖金来自主场调整),而如果西雅图来了,巨人将获得西雅图必须长途跋涉的62点Elo奖励。
Margin-of-victory Multiplier — All the adjustments above would be sufficient if were judging teams based solely on wins and losses. However we want the model to take into account how a team won — whether they dominated their opponents or simply squeaked past them. Therefore there is a multiplier that increases the amount of Elo points a team gets based on how they won. The formula for this multiplier is below.
获胜保证金乘数—如果仅根据获利和亏损来评估团队,则上述所有调整就足够了。 但是,我们希望该模型考虑到一支球队是如何获胜的-无论他们是统治对手还是干脆越过对手。 因此,存在一个乘数,该乘数会根据团队的获胜方式增加其获得的Elo点数。 该乘数的公式如下。
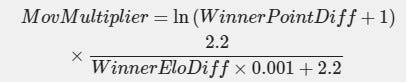
If your wondering how specific numbers like add 55 Elo points for the Home-Field Advantage came to be, well it’s because every point in a NFL game is equal to 25 Elo points in the model. As I said before, teams that play at home win by 2.2 points more on average. 2.2 x 25 =55, this is how the model finds out how much it should add for each adjustment.
如果您想知道像这样的特定数字如何为Home-Field Advantage增加55个Elo点,那是因为NFL游戏中的每个点都等于模型中的25个Elo点。 就像我之前说的,主场比赛的球队平均多赢2.2点。 2.2 x 25 = 55,这是模型找出每次调整应添加的数量的方式。
季前评分 (Preseason Ratings)
One thing about the Elo model that should be known is that it’s always continuous. The Elo’s for each team don’t reset after each season, instead the Elo ratings get carried over to next season. To account for offseason changes that may include draft picks, free agency, trades, and coaching changes there is a preseason adjustment for the Elo ratings.
关于Elo模型应该知道的一件事是它总是连续的。 每个团队的Elo值在每个赛季后都不会重置,而是将Elo评级延续到下一个赛季。 要考虑可能包括选秀权,自由球员,交易和教练变更在内的休赛期变更,请对Elo评分进行季前调整。
This adjustment uses two factors. The first is reverting a team’s end of season Elo one-third of the way toward a mean of 1505. The second involves using Vegas win totals for next season.
此调整使用两个因素。 首先是将一支球队的Elo赛季结束时的三分之一恢复到平均1505。第二是使用下个赛季的拉斯维加斯总胜率。
What forms a team’s Elo rating for the start of next season is one-third weight given to regressed Elo and two-thirds weight given to Vegas-wins.
在下个赛季开始时构成球队Elo评分的因素是,回归Elo占三分之一的权重,而对拉斯维加斯胜利则占三分之二的权重。
准确性 (Accuracy)
Taking a look back at the Dallas vs New York example, Dallas’s Elo rating was 1650 and New York’s was 1400. The difference between the two is 250. If we divide 250/25 we get 10. What this means in the model is that it predicts Dallas to win by 10 points against New York. This is how point spreads are predicted for each game. The model takes the Elo difference and divides it by 25, because like I mentioned before, every point in an NFL game equals 25 Elo points in the model.
回想一下达拉斯与纽约的例子,达拉斯的Elo评分为1650,纽约为1400。两者之差为250。如果我们将250/25除以10,则该模型的含义是预测达拉斯对阵纽约时将赢得10分。 这就是每个游戏预测点差的方式。 该模型采用Elo差异并将其除以25,因为就像我之前提到的那样,NFL游戏中的每个分数等于模型中的25 Elo分数。
Predicted point spreads are a great way to evaluate prediction accuracy by comparing it the actual score differential of each game. That’s exactly how FiveThirtyEight judges the accuracy of their models. Using Mean Squared Error, the model finds the difference between the predicted point spread and actual, and squares the errors to give a larger penalty for bad misses. The lower the error the better.
预测点差是通过比较每个游戏的实际得分差异来评估预测准确性的好方法。 这正是FiveThirtyEight判断其模型准确性的方式。 使用均方误差,模型可以找到预测点扩散与实际值之间的差异,并对误差进行平方,以对不良后果给予更大的惩罚。 误差越小越好。
Mean Squared Error Formula:
均方误差公式:
(Predicted Point Spread - Actual Score Diff)² = MSEExample: (10 - 7)² = 9
FiveThirtyEight also evaluates their models using Brier Scores. Instead of point spreads, Brier Scores use win probabilities. In our Dallas example the model gave them a win probability of 80%. At 80% the model is very confident Dallas will win and if they do the Brier Score will be low (Lower Brier scores are better because they mean the prediction was closer to being correct). However, if New York wins with only a 20% win probability, that means our model was very wrong in predicting the outcome of this game and the Brier Score will be quite high.
FiveThirtyEight还使用Brier分数评估他们的模型。 Brier分数使用获胜概率代替点差。 在我们的达拉斯示例中,模型为他们提供了80%的获胜概率。 模型达到80%时,达拉斯很有信心赢得胜利,如果他们做到了,则Brier得分会很低(较低Brier得分会更好,因为它们意味着预测更接近于正确)。 但是,如果纽约仅以20%的获胜概率获胜,则意味着我们的模型在预测这场比赛的结果时非常错误,并且Brier得分将非常高。
Brier Score Formula:
石蜡分数公式:
(Win Probability — Outcome of Game)² = Brier ScoreExample:
(0.80 — 1)² = 0.04 #If Dallas wins the game
(0.80 — 0)² = 0.64 #If Dallas loses the game
最新版本 (Latest Version)
Over the years, FiveThirtyEight’s Elo prediction model has been continuously updated with new adjustments to further improve prediction accuracy in these two metrics. In 2019 their latest version came out and it performed much better then previous versions. The graphs below show the difference between the newest version and the older versions in both accuracy metrics. This increase in accuracy can be contributed to one more adjustment in their latest model that is their biggest and maybe their most important that we haven’t discussed.
多年来,FiveThirtyEight的Elo预测模型已通过新的调整不断进行更新,以进一步提高这两个指标的预测准确性。 在2019年,他们的最新版本问世,其性能比以前的版本好得多。 下图显示了这两个准确性指标中最新版本与旧版本之间的差异。 准确性的提高可促成他们最新模型的又一次调整,这是他们最大,甚至是我们尚未讨论的最重要模型。
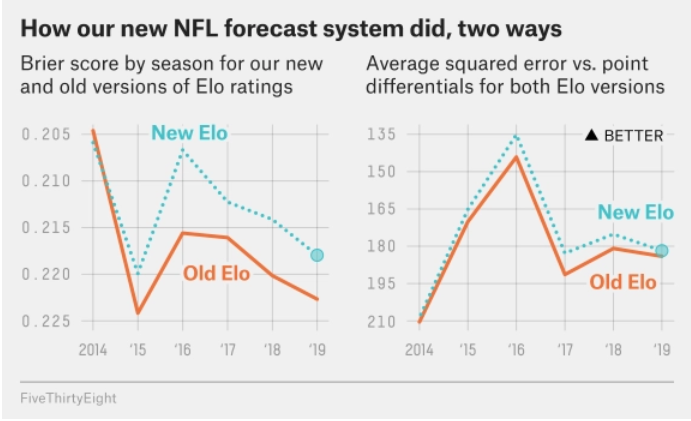
四分卫调整 (The Quarterback Adjustment)
In 2019 FiveThirtyEight added an adjustment for the game’s most important position. Here’s how it works:
在2019年FiveThirtyEight增加了对游戏最重要位置的调整。 运作方式如下:
- Every team and quarterback in the NFL get a VALUE rating based on their recent performances. This VALUE rating either increases or decreases based on the quarterback’s performance in games. NFL中的每支球队和四分卫都根据他们最近的表现获得VALUE评分。 根据四分卫在游戏中的表现,此VALUE评级会增加或减少。
How the model evaluates how well a quarterback performed in a game is through this formula: -2.2 * Pass Attempts + 3.7 * Completions + (Passing Yards / 5) + 11.3 * Passing TDs — 14.1 * Interceptions — 8 * Times Sacked — 1.1 * Rush Attempts + 0.6 * Rushing Yards + 15.9 * Rushing TDs
该模型如何通过以下公式评估四分卫在游戏中的表现: -2.2 *传球尝试+ 3.7 *补全+(传球次数/ 5)+ 11.3 *传球TD-14.1 *拦截-8 *被解雇的时间-1.1 *急速尝试+ 0.6 *急速码+ 15.9 *急速TD
- The formula above is a quarterback’s Game_VALUE. After every game the quarterback’s VALUE is either increased or decreased based on their Game_VALUE. This is done by contributed the updated quarterback VALUE to 90% it’s old VALUE, and 10% it’s Game_VALUE (i.e., VALUE_Rating_New = 0.9 * VALUE_Rating_Old + 0.1* Game_VALUE) 上面的公式是四分卫的Game_VALUE。 每场比赛之后,四分卫的VALUE会根据其Game_VALUE增减。 这是通过将更新的四分卫VALUE贡献到旧的VALUE的90%和Game_VALUE的10%(即VALUE_Rating_New = 0.9 * VALUE_Rating_Old + 0.1 * Game_VALUE)来完成的
- This VALUE is also adjusted for opposing defensive quality by computing for team QB VALUE allowed. This is done by subtracting the league average from the VALUE an opponent usually gives up per game, and using that to adjust for a QB’s performance for the game in question. For example, if the opposing team usually gives up a QB VALUE 5 points higher than the average team, we would adjust the other teams individual QB’s performance upward by 5 points of VALUE to account for the easier opposing defense. 还可以通过计算允许的球队QB VALUE来调整此VALUE,以提高防守质量。 这是通过从对手通常每场比赛放弃的价值中减去联赛平均值,然后使用该平均值来调整所讨论游戏的QB表现来实现的。 例如,如果对方球队通常将QB VALUE比平均球队高5分,那么我们会将其他球队个人QB的表现上调VALUE 5点,以说明对手防守更容易的情况。
- The QB VALUE represents what we’d expect a quarterback to produce against a passing defense of average quality in the next start. To convert this VALUE rating to Elo, the VALUE can be multiplied by 3.3 to get the number of Elo points a QB is expected to be worth compared with an undrafted rookie replacement. QB值代表我们期望四分卫在下一次比赛中产生的对抗平均质量的过时防守。 要将VALUE等级转换为Elo,可将VALUE乘以3.3以得到QB与未起草的新秀替换相比预期值得的Elo点数。
- To get an idea of the different VALUE ratings a quarterback can have, below is a table of the top 10 quarterbacks in VALUE in the 2019 season. 为了了解四分卫可以拥有的不同VALUE评级,下表是2019赛季VALUE前十名的四分卫的表格。
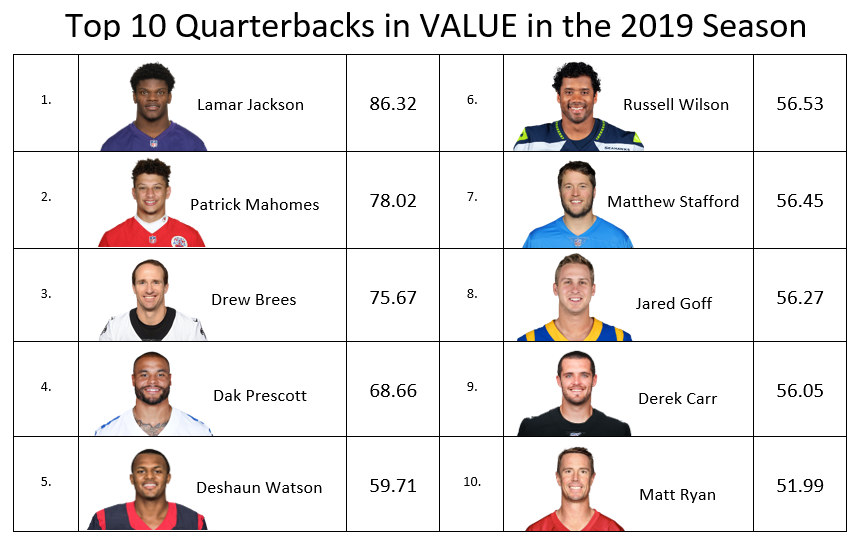
The quarterback Elo adjustment is applied before each game by comparing the starting QB’s VALUE rating with the team’s VALUE rating and multiplying by 3.3.
通过将起始QB的VALUE等级与球队的VALUE等级进行比较并乘以3.3,在每场比赛之前应用四分卫Elo调整。
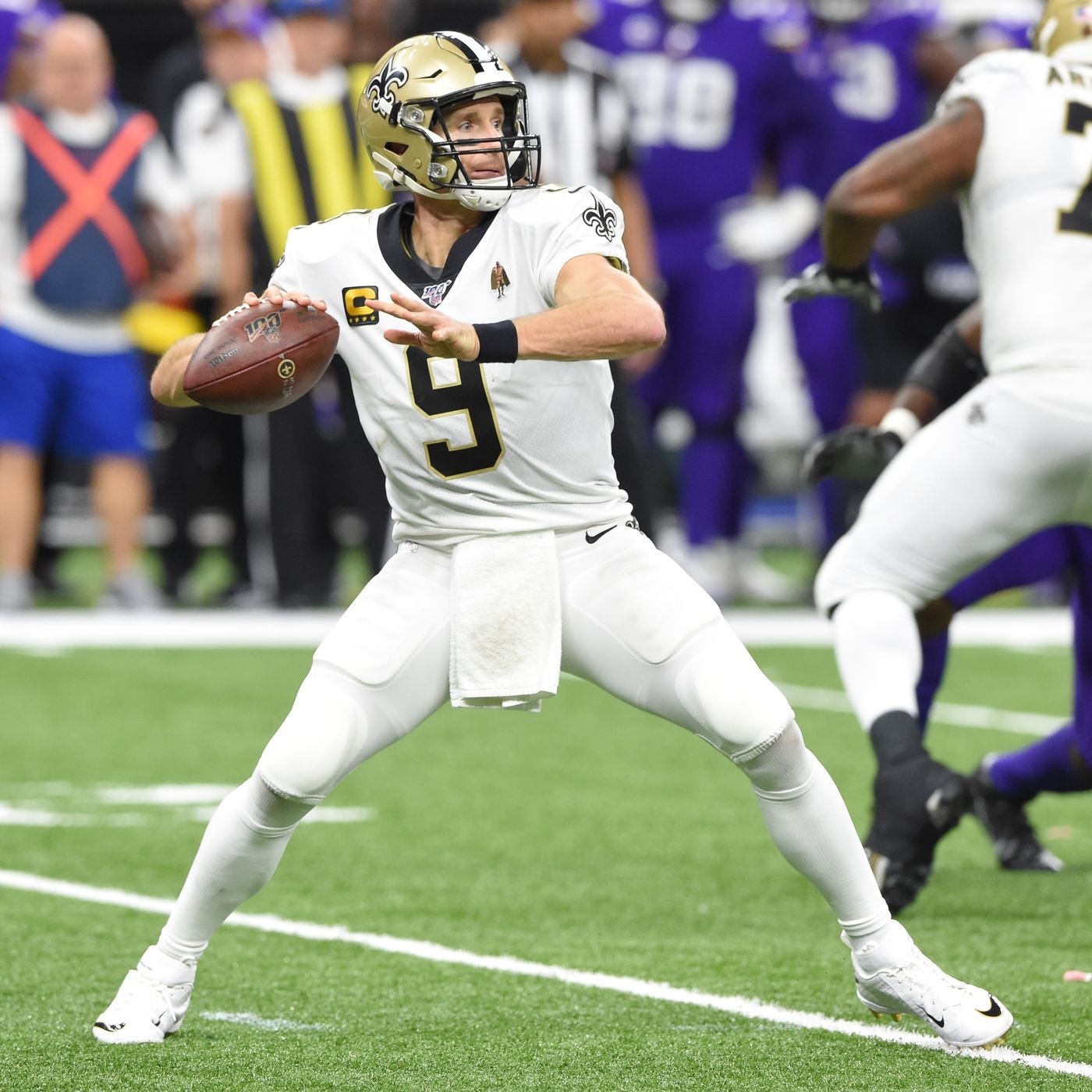
Example: When Drew Brees was injured midway through the 2019 season, he had a VALUE rating of 75. The New Orleans Saints team VALUE rating was 78, and backup Teddy Bridgewater had a personal rating of 45. So when adjusting the Saints Elo for their next game with Bridgewater starting instead of Brees, we would have applied an adjustment of 3.3 * (45–78) = -109 to New Orleans’ base Elo rating of 1639 heading into its Week 4 game against the Cowboys. This effectively would have left the Saints as a 1530 Elo team with Bridgewater under center (before applying adjustments for home field, travel and rest), dropping New Orleans’s win probability from 60% to 44% for the game despite playing at home. In cases like these, the QB adjustment can have a massive effect!
示例:当Drew Brees在2019赛季中段受伤时,他的得分为75。新奥尔良圣徒队的得分为78,替补泰迪·布里奇沃特的得分为45。因此,在调整Saints Elo时在下一个以布里奇沃特而不是布雷斯开始的比赛中,我们将对新奥尔良的基本Elo评分1639进行3.3 *(45–78)= -109的调整,以进入第4周对阵牛仔的比赛。 这实际上会使圣徒成为1530年Elo球队,而布里奇沃特(Bridgewater)居中(在对本垒打,出差和休息进行调整之前),尽管在主场打球,新奥尔良的比赛获胜概率从60%降至44%。 在这种情况下,QB调整会产生巨大影响!
Just like how a team’s preseason rating is adjusted, so are individual quarterbacks. When the offseason rolls around, quarterbacks have their final VALUE rating from the end of the season reverted toward the average QB VALUE by one-fourth before the following season.
就像团队的季前评分如何调整一样,个人四分卫也是如此。 当休赛期临近时,四分卫将其从本赛季末开始的最终VALUE等级恢复为下一个赛季之前四分之一的平均QB VALUE。
For rookies making their starting debuts, we assign them initial ratings based on draft position. An undrafted rookie is always assigned a rating of zero for his first start. The first overall pick, by comparison, gets a VALUE rating of 35 before his first start.
对于首次亮相的新秀,我们根据选秀位置为其分配初始评级。 未起草的新秀在首次开始时总是被评为零。 相比之下,首个总体选秀权在他首次出发前的得分为35。
You should now have a higher quality understanding of how the FiveThirtyEight model works. It is a simple but effective model to predict the outcome of games, win probabilities, and their point spreads.
现在,您应该对FiveThirtyEight模型的工作原理有更高的了解。 这是一个简单但有效的模型,可以预测游戏的结果,获胜概率及其点差。
However, the model can be improved even further, and improve it I did! I have found other adjustments that the people at FiveThirtyEight did not and ended up improving the model greatly.
但是,该模型可以进一步改进,并且可以改进它! 我发现了FiveThirtyEight员工没有进行的其他调整,最终极大地改进了模型。
改进之处 (The Improvements)
When trying to figure out how to improve FiveThirtyEight’s model, I had to think about what aspects of the game did they not adjust for. As we discussed before they already have adjustments for: home field, bye weeks, playoff favorites, travel distance, and changes at starting quarterback. But diving deeper into the game of football I was able to find 3 new adjustments that heavily improved the model.
当试图找出如何改进FiveThirtyEight的模型时,我不得不考虑他们没有适应游戏的哪些方面。 正如我们之前讨论的那样,他们已经针对以下方面进行了调整:主场,再见周,季后赛最爱,旅行距离以及四分卫开始时的变化。 但是深入研究足球比赛,我发现了3个新的调整,这些调整极大地改善了模型。
1.团队比赛的哪一天 (1. What Day a Team Plays On)
Kirk Cousins, the quarterback of the Minnesota Vikings is the inspiration for this adjustment. Cousins is famous for playing terribly in prime time games (Monday night. Thursday night, and Sunday night games). In fact his record in these games is 6–13 despite playing on an above average team.
明尼苏达维京人队的四分卫柯克·考辛斯是这项调整的灵感来源。 表兄弟因在黄金时段比赛(周一晚上,周四晚上和周日晚上的游戏)中表现出色而闻名。 实际上,尽管他的战绩高于平均水平,但他在这些比赛中的战绩为6-13。
This got me thinking, in prime time games on national television when the whole world is watching, do teams play better or worse then when playing on a normal Sunday afternoon. Well when diving into the stats over the last 10 years this is what I found…
这让我想,在全世界观看比赛的国家电视台黄金时段比赛中,与在正常的周日下午比赛时相比,球队的表现会好还是坏。 好吧,在过去十年中深入研究统计数据时,这就是我发现的…
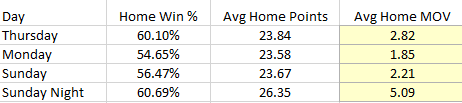
As you can see from the table above in Thursday and Sunday Night games, the home team has a greater chance of winning by nearly 5% more. These teams also increase their average margin of victory in these games by around 1 point on Thursday night and about 2.5 points on Sunday night, thus proving at home, teams typically play better in prime time games. Therefore, the adjustment that is added to our improved model is to add 25 Elo points to the home team on Thursday night games, and add 50 Elo points to the home team on Sunday night games.
从上周四和周日晚上的比赛表中可以看出,主队获胜的机会更大,接近5%。 这些球队在周四晚上将这些比赛的平均获胜幅度提高约1点,在周日晚上将其增长约2.5点,因此在国内证明,球队通常在黄金时段比赛中表现更好。 因此,对我们改进后的模型进行的调整是在周四晚上的比赛中为主队增加25个Elo点,在周日晚上的比赛中给主队增加50个Elo点。
2.分区比赛 (2. Divisional Games)
In each division in the NFL there are 4 teams. These teams play each other twice in the regular season. Since teams in the same division see each other more often than other teams, they get the advantage of playing them once, adjusting for them, then playing them again. This would ensue that divisional games should be much closer than non divisional games since these teams have already seen how the other plays, and they can prepare for it.
在NFL的每个部门中,有4支球队。 这些球队在常规赛中互相比赛两次。 由于同一个部门中的团队比其他团队更经常见到对方,因此他们获得了一次比赛,为他们进行调整,然后再进行比赛的优势。 这将确保分区比赛比非分区比赛更加接近,因为这些团队已经了解了其他球队的比赛方式,并且可以为此做准备。
The table below shows the difference between divisional games and non-divisional games over the last 10 years.
下表显示了过去10年中分区游戏与非分区游戏之间的差异。

As seen from the table above, in divisional games the home team has a lower chance to win than non-divisional games by nearly 3%. Their average margin of victory also decreases by a point when playing a team in their division, proving that divisional games are much closer and harder to predict then non-divisional games. To adjust for the closer divisional games, our improved model reduces the team with the higher amount of Elo points by 25 to minimize the overall difference in Elo between the two division teams.
从上表可以看出,在分区比赛中,主队获胜的机会比非分区比赛低近3%。 当与他们的部门比赛时,他们的平均获胜率也会降低一个点,这证明部门比赛比非部门比赛更加紧密和难以预测。 为了适应更接近的分区比赛,我们改进的模型将拥有更高Elo点数的团队减少了25个,以最大程度地减少两个分区团队之间的Elo总体差异。
3.拉斯维加斯点差 (3. Vegas Point Spreads)
As I discussed before, FiveThirtyEight’s model is famous because it competes with the ones in Vegas. In fact when comparing their model’s predicted point spreads with Vegas’s predicted point spreads, in about 80% of games the difference between the two is a mere field goal (3 points or less). But the disadvantage that the Elo model has is that it’s not up to date like the Vegas model.
正如我之前讨论的那样,FiveThirtyEight的模型很出名,因为它可以与拉斯维加斯的模型竞争。 实际上,当将他们的模型的预测点差与维加斯的预测点差进行比较时,在大约80%的游戏中,两者之间的差异仅仅是一个射门得分(3分或更少)。 但是Elo模型的缺点是它不像Vegas模型那样是最新的。
When Vegas rolls out their point spreads they are imputing every factor they can find; injuries, weather, last second starting change, etc. The Elo model doesn’t do this. Most of it’s adjustments you can find the info a week before. The only up to date adjustment is the quarterback adjustment that applies when there is a quarterback change, but for ever other position an adjustment does not exist.
当Vegas推出其点差时,他们将尽其所能找到所有因素。 人身伤害,天气,最近的第二次开始更改等。Elo模型不执行此操作。 大多数调整都是您可以在一周前找到的信息。 唯一最新的调整是四分卫调整,当四分卫发生变化时适用,但对于其他任何位置,都不存在调整。
If in about 80% of games the difference between the two point spreads is about 3 points, then what happened with the other 20%? In my conclusion I believe there may have been an injury besides the quarterback position, or an unseen circumstance that the Elo model couldn’t adjust for, that may have occurred to make the difference between the two point spreads greater than 3. This is where our 3rd adjustment takes place.
如果在大约80%的游戏中,两点差之间的差约为3点,那么其他20%怎么办? 在我的结论中,我相信除了四分卫位置之外,还可能有受伤的情况,或者是Elo模型无法适应的看不见的情况,可能是使两点之间的差值大于3。我们进行了第三次调整。
Since our model doesn’t adjust for these scenarios, I decided to get a little help from the Vegas model. Therefore, if the difference between our model’s predicted point spread and Vegas’s predicted point spread is greater than 3, then we adjust our predicted point spread to match Vegas’s. For example, if our model predicts the Cowboys to win by 10, but Vegas predicts they win by 6, then we adjust the Elo’s for the two teams so our predicted point spread is then 6.
由于我们的模型无法适应这些情况,因此我决定从Vegas模型中获得一些帮助。 因此,如果模型的预测点差与Vegas的预测点差之差大于3,则我们将调整我们的预测点差以匹配Vegas的点。 例如,如果我们的模型预测牛仔队获胜10场,而维加斯预测牛仔队获胜6场,则我们调整两支球队的Elo,因此我们的预测分差为6。
So like in the example above, Dallas’s Elo was 1650 and New York’s was 1400. The difference in Elo is 250 and 250/25 =10. Our model predicts the point spread to be 10, but we want it to match Vegas at 6. Therefore, we reduce Dallas’s Elo from 1650 to 1550.
因此,就像上面的示例一样,达拉斯的Elo为1650,纽约为1400。Elo之差为250和250/25 = 10。 我们的模型预测点扩散为10,但我们希望点扩散与维加斯匹配,为6。因此,我们将达拉斯的Elo从1650降低到1550。
1550–1400 = 150, 150/25 = 6, our now current predicted point spread (This also reduces Dallas’s predicted win probability from 80% to 70%).
1550–1400 = 150,150/25 = 6,我们现在的当前预测点差(这也将达拉斯的预测胜出概率从80%降低到70%)。
我们改进的模型本赛季的表现如何 (How Our Improved Model Did This Season)
2019 was a bit of an unpredictable NFL season. This is backed by the fact that in both of FiveThirtyEight’s newest and older Elo model versions, both accuracy metrics (Brier Scores, Mean Squared Error) took a dip from it’s previous years. But how well did our improved model do in this unpredictable NFL season? By comparing all 3 models in both accuracy metrics, we can determine which model performed the best in the 2019 season.
2019年是不可预测的NFL赛季。 这是由以下事实支持的:在FiveThirtyEight的最新和较旧的Elo模型版本中,这两个准确性指标(Brier分数,均方误差)都比前几年有所下降。 但是我们改进后的模型在这个不可预测的NFL赛季表现如何? 通过将两个模型的两个准确性指标进行比较,我们可以确定哪个模型在2019赛季中表现最好。
石蜡分数: (Brier Scores:)
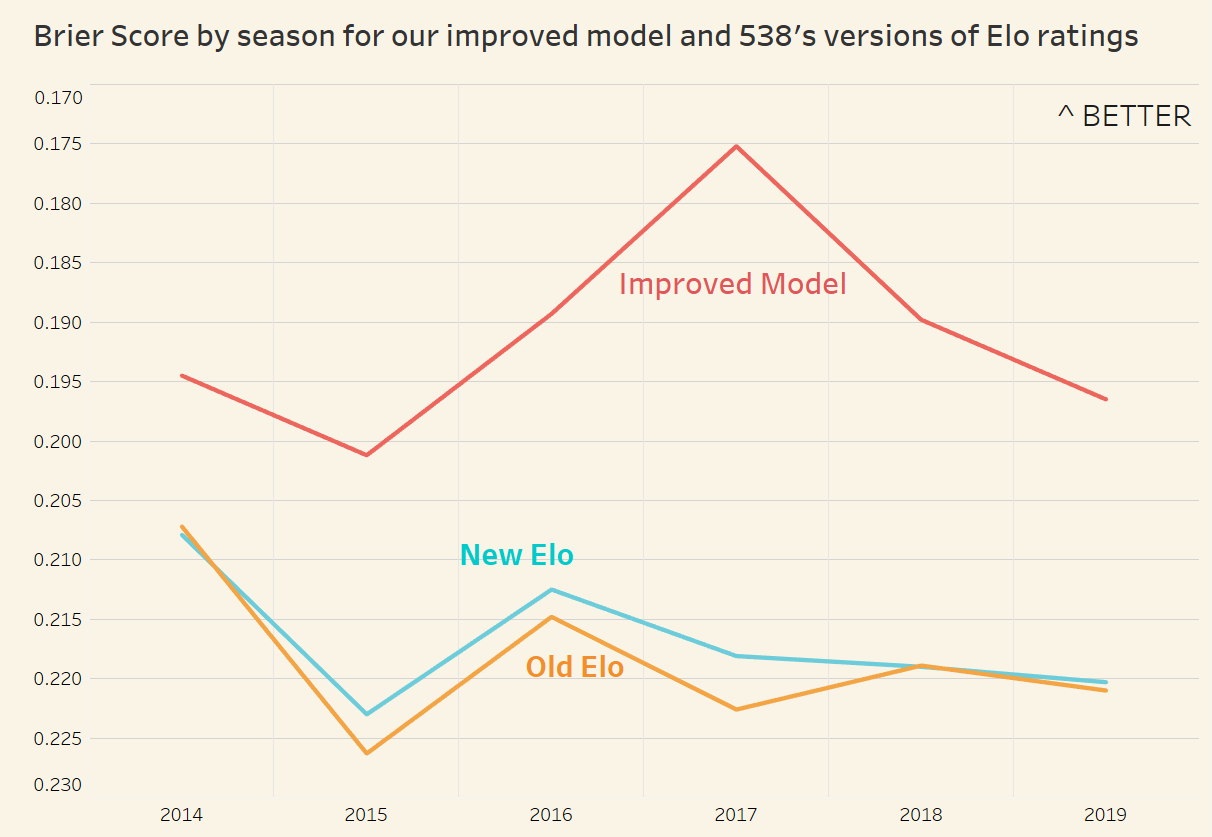
If you remember the lower the Brier Score the better, and our improved model was able to lower the Brier Score significantly. In 2019 New Elo had an average Brier Score of 0.219, and our improved model had an average of 0.196, showing in 2019 our model was able to predict the win probabilities of team’s much better than both Elo versions. But it wasn’t just 2019. If you look at the graph above, over the last 5 years our improved model performed better than both Elo versions every year.
如果您还记得Brier分数越低越好,并且我们改进的模型能够显着降低Brier分数。 在2019年,新Elo的平均Brier得分为0.219 ,而改进后的模型的平均得分为0.196 ,这表明在2019年,我们的模型能够预测团队的获胜概率远胜于两个Elo版本。 但这不只是2019年。如果您查看上面的图表,在过去的5年中,我们改进的模型每年的表现都优于两个Elo版本。
Using Brier Scores, let’s take a look at how each model’s accuracy evolved over time in the regular season. As you can see from the graph below in weeks 3, 4, and 9 our model did worse than the Elo versions. But for the rest, our improved model was able to outperform. This means in 14/17 weeks our improved model performed the best. This resulted in the decrease of the overall average Brier Score for the 2019 season.
使用Brier分数,让我们看一下常规季节每个模型的准确性如何随时间变化。 从下面的图表中可以看到,在第3、4和9周中,我们的模型比Elo版本差。 但是对于其余部分,我们改进的模型能够胜过其他公司。 这意味着在14/17周内,我们改进的模型表现最佳。 这导致2019赛季总体平均Brier得分下降。
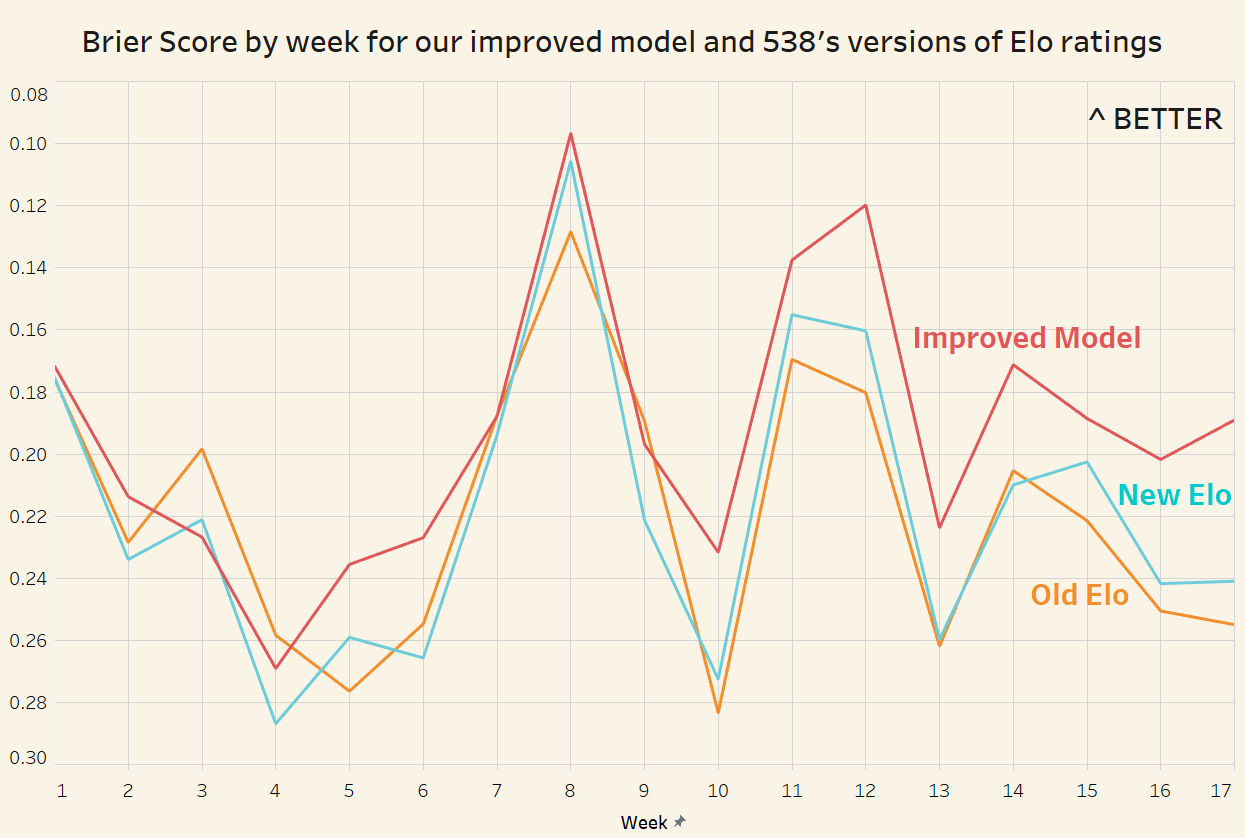
均方误差: (Mean Squared Error:)
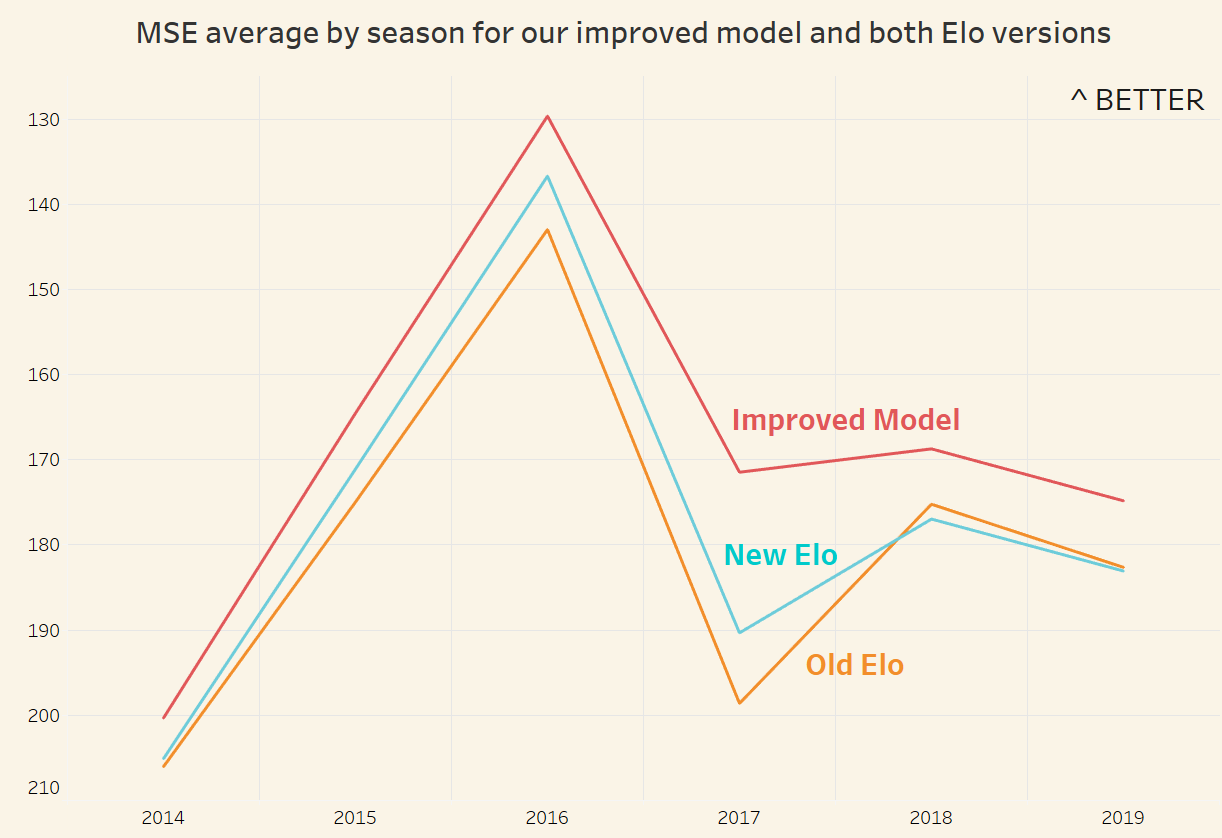
Similarly to Brier Scores, our improved model out performed both Elo versions every year in reference to Mean Squared Error. In 2019 New Elo had an average MSE of 183, while our improved model had an average of 174, showing in 2019 our model was able to predict the point spreads of games much better than both Elo versions.
与Brier分数类似,我们改进的模型每年参考均方误差执行两种Elo版本。 在2019年,新Elo的平均MSE为183 ,而改进后的模型的平均MSE为174 ,这表明在2019年,我们的模型能够比两个Elo版本更好地预测游戏的点差。
最伟大的流行曲 (Greatest Hits and Misses)
You’ve already seen how our improved model has done better than the Elo versions on a season and week to week basis. In theory, the next part is to show what specific games did our improved model increase in accuracy the most.
您已经看到我们的改进模型在每个季节和每周的基础上都比Elo版本做得更好。 从理论上讲,下一部分将展示我们改进的模型在最大程度上提高了哪些特定游戏的准确性。
Down below are two charts. The first displays our improved models best picks relative to the newest Elo version, the second displays the worst.
下面是两个图表。 第一个显示相对于最新的Elo版本,我们改进的模型最佳选择,第二个显示最差的模型。
(OLD column refers to FiveThirtyEight’s newest Elo models predicted winning percentage for the team that won. NEW column is our improved models predicted winning percentage for that same team).
(旧列是指FiveThirtyEight最新的Elo模型预测获胜球队的获胜百分比。NEW列是我们改进的模型预测相同球队的获胜百分比)。
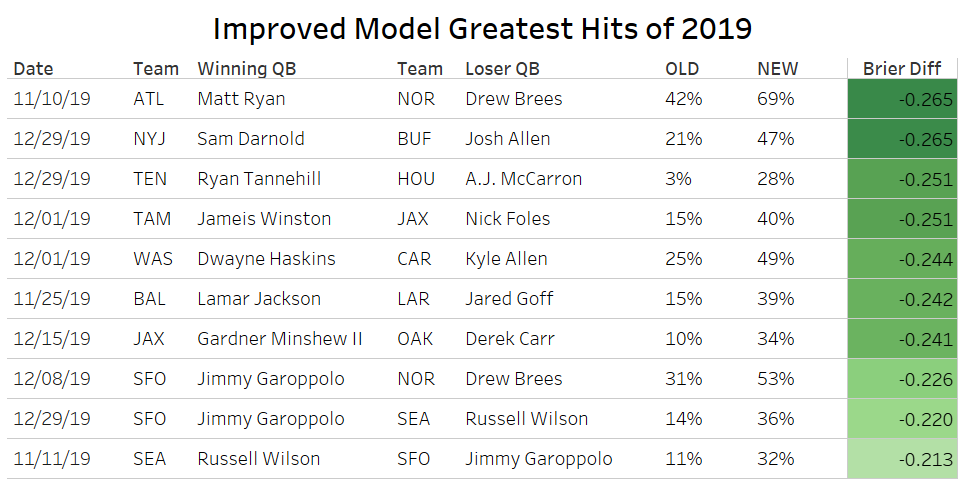
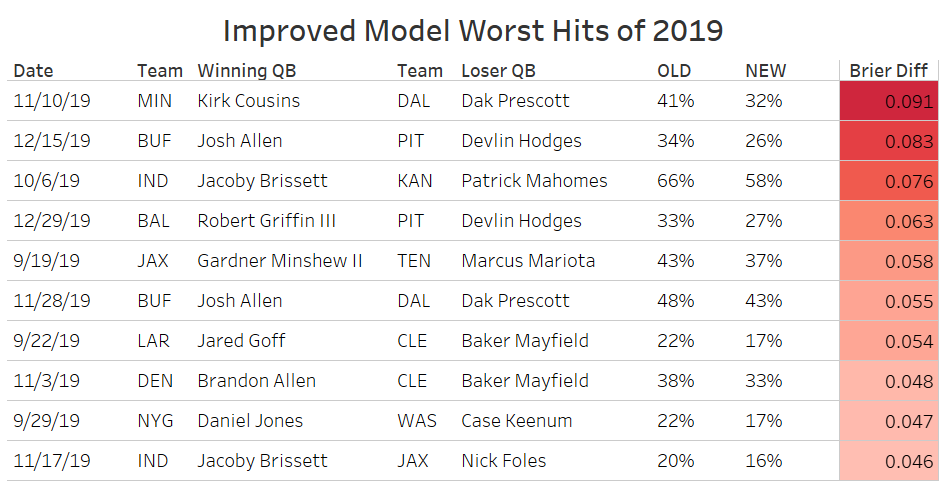
FiveThirtyEight的NFL预测游戏 (FiveThirtyEight’s NFL Forecast Game)
Every year FiveThirtyEight host an NFL forecast competition to see if anyone can beat their Elo models in forecasting NFL games. Yearly, thousands sign up to prove that they know more about football than FiveThirtyEight and anyone else.
每年FiveThirtyEight都会举办一次NFL预测比赛,以查看是否有人可以在预测NFL游戏中击败他们的Elo模型。 每年,成千上万的人报名参加,证明他们比FiveThirtyEight和其他任何人都对足球了解更多。
The game works by using Brier Scores. Every week of the NFL season (including playoffs) players decide which team will win by giving that team a winning percentage. After each NFL game finishes, players will either gain or lose points based on whether they picked the winning team and how confident they were that they would prevail. The higher a win probability you assign a team, the more points you can earn — but also the more you can lose if you were over confident.
该游戏通过使用Brier分数来工作。 在NFL赛季的每个星期(包括季后赛),球员都通过给该队一个胜率来决定哪支球队获胜。 在每场NFL游戏结束后,玩家将根据是否选择获胜的球队以及他们对自己能否取得胜利的信心来获得或减少得分。 您分配一支球队的获胜概率越高,您可以获得的积分就越多,但如果您过分自信,则损失的钱也就越多。
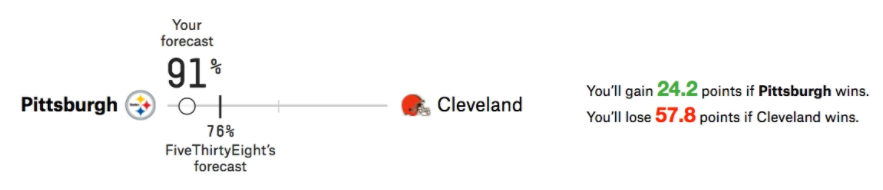
To further demonstrate that our improved model can forecast NFL games better than FiveThirtyEight, I decided to participate in their game to see how many points my improved model would of accumulated if we indeed played in the 2019 season.
为了进一步证明我们的改进模型比FiveThirtyEight更好地预测NFL游戏,我决定参加他们的比赛,以查看如果我们确实在2019赛季打球,我的改进模型将累积多少点。
In 2019 over 15,000 players signed up to play FiveThirtyEight’s NFL forecast game. At the end of the season FiveThirtyEight’s model had accumulated 773.7 points, good enough to be in the 97th percentile and in 514th place. This means their model was able to predict NFL games better then 97% of those that played. In comparison, Griffin Colaizzi who came in 1st place finished with 1,126.2 points and was in the 99th percentile.
在2019年,超过15,000名球员报名参加了FiveThirtyEight的NFL预测游戏。 在本赛季结束时,FiveThirtyEight的模型累积了773.7分,足以在第97个百分位和第514位排名。 这意味着他们的模型能够预测NFL比赛,胜过之前比赛的97%。 相比之下,第一名的格里芬·科莱兹(Griffin Colaizzi)以1,126.2分的成绩排名第99位。
Question is, was our improved model able to beat FiveThirtyEight’s score, and did it come even close to Griffin’s.
问题是,我们改进的模型是否能够超过FiveThirtyEight的得分,并且甚至接近Griffin的得分。
Down below is a weekly chart of the net points our improved model gained each week in the NFL forecast game.
下面是每周的图表,这些图表是我们改进后的模型在NFL预测游戏中每周获得的净积分。
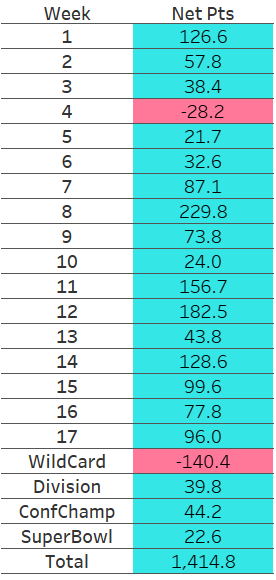
In all but 2 weeks our improved model stayed in the positive for net points, and was able to finish the season with a total score of 1,414.8 points. Not only did we nearly double FiveThirtyEight’s points, but we blew Griffin’s total out of the water by gaining almost 300 more points than he did. This means if our improved model had competed in the forecast game during the season, not only would we have come in 1st place out of nearly 15,000 competitors, but it wouldn’t even have been close.
除了2周外,我们改进的模型均保持净积分为正,并以1,414.8分的总成绩完成了赛季。 我们不仅使FiveThirtyEight的点数几乎翻了一番,而且使Griffin的总点数比他增加了近300倍,这使他从水里滑落了。 这意味着,如果我们改进的模型在本赛季中参加了预测比赛,那么我们不仅会在将近15,000个竞争对手中名列第一,而且甚至不会接近。
季节模拟 (Season Simulations)
The reason the Elo model is so great is because most of the info needed to predict games can be found months before when the schedule for next season comes out. Since we are days away from the start of the season, and we now understand how the Elo ratings update as the schedule plays out, we have the info we need and can predict what will happen over the entire 2020 season.
Elo模型之所以如此出色,是因为预测下赛季所需的大多数信息都可以在下个赛季的时间表出台前几个月找到。 由于我们距离本赛季开始只有几天的时间,并且我们现在了解随着时间表的进行,Elo评分会如何更新,因此我们可以获得所需的信息,并可以预测整个2020赛季将会发生什么。
The NFL season isn’t always definite, teams that should typically blow out others teams sometimes lose. This infers there’s randomness in an NFL season. To integrate this randomness into our model, we use the Monte Carlo Method and simulate the 2020 NFL season 100,000 times, tracking how often each simulated universe yields a given outcome for each team.
NFL赛季并不总是确定的,通常应该击败其他球队的球队有时会输掉。 这说明NFL赛季存在随机性。 为了将这种随机性整合到我们的模型中,我们使用蒙特卡洛方法并模拟100,000次2020 NFL赛季,追踪每个模拟宇宙为每个团队产生给定结果的频率。
In the end the simulations will show a team’s expected Elo points, point differential, full-season record and it’s odds of winning it’s division, making the playoffs, getting a first round bye, and even winning the Super Bowl.
最后,模拟将显示团队的预期Elo积分,积分差,全赛季战绩,并有可能赢得分区冠军,进入季后赛,获得第一轮再见,甚至赢得超级碗冠军。
Find you favorite team down below, and see what our model predicts will happen for your team next season.
在下方找到您最喜欢的球队,然后看看我们的模型预测下赛季您的球队会发生什么。
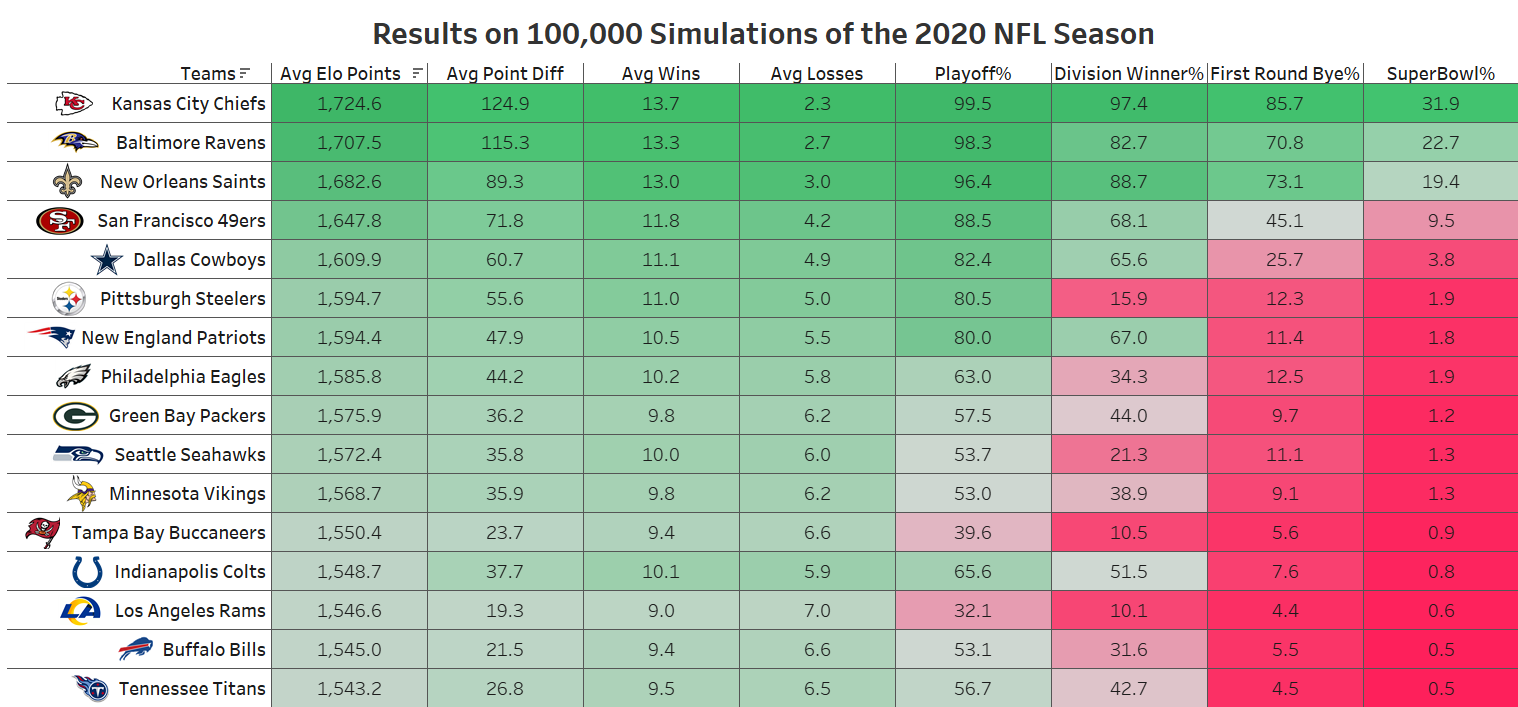
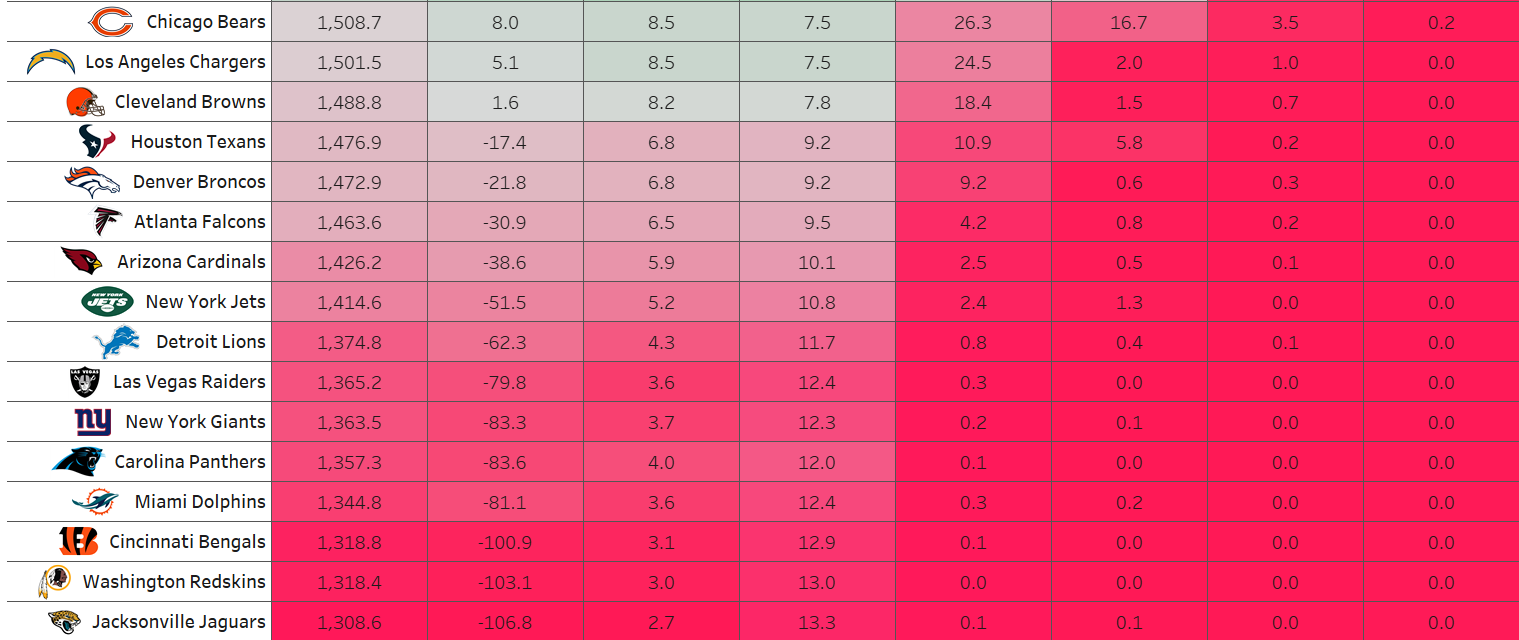
结论 (Conclusion)
FiveThirtyEight’s Elo model is simple but effective. Through Elo ratings it’s easy to tell the strength of any given team and predict a winner. The adjustments that come along with the model help those predictions become even more accurate by dealing with situations in and outside of the direct game.
FiveThirtyEight的Elo模型简单但有效。 通过Elo评分,很容易分辨出任何给定团队的实力并预测获胜者。 该模型附带的调整功能通过处理直接游戏内部和外部的情况,使这些预测变得更加准确。
We were able to improve their Elo model by recreating it and adding a few adjustments of our own. But can we improve it even further? Absolutely! Football is not stagnant, it is ever changing and new patterns and play styles will emerge that will change how the game is played. This is why Vegas’s models continue to be some of the best, they always change based on how the game has. By doing the same, the Elo model can compete with some of Vegas’s best and may have the ability to even surpass.
我们能够通过重新创建模型并添加一些自己的调整来改进其Elo模型。 但是我们可以进一步改善它吗? 绝对! 足球并没有停滞不前,而是不断变化,新的格局和比赛方式将出现,从而改变比赛的方式。 这就是维加斯模型仍然是最好的模型的原因,它们总是根据游戏的方式进行更改。 通过这样做,Elo模型可以与Vegas的一些最佳产品竞争,甚至有可能超越。
谢谢 (Thank You)
I would like to give a short paragraph to say thank you to the guys at FiveThirtyEight. They have done an excellent job in providing details on their models that allowed me to recreate them. I discussed most of what the model incorporates in this article, but if you want to read the details for yourself here’s a link to their webpage: How Our NFL Predictions Work. If you would also like to play and compete in their NFL forecast game, I will leave a link to that as well: How To Play Our NFL Predictions Game.
我想简短地对在FiveThirtyEight的家伙们表示感谢。 他们在提供模型细节方面做得非常出色,这使我能够重新创建它们。 我讨论了该模型在本文中包含的大部分内容,但是如果您想自己阅读详细信息,请访问他们的网页链接: 我们的NFL预测如何工作 。 如果您还想参加他们的NFL预测游戏并参加比赛,我也将留下一个链接: 如何玩我们的NFL预测游戏 。
翻译自: https://medium.com/the-sports-scientist/improving-a-famous-nfl-prediction-model-1295a7022859
数学模型预测模型